
Speaker "Noam Bressler" Details Back
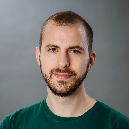

-
Name
Noam Bressler
-
Company
Deepchecks
-
Designation
Manager
Topic
How to Properly Test ML Models & Data
Abstract
Automatic testing for ML pipelines is hard. Part of the executed code is a model that was dynamically trained on a fresh batch of data, and silent failures are common. Therefore, it’s problematic to use known methodologies such as automating tests for predefined edge cases and tracking code coverage. In this talk we’ll discuss common pitfalls with ML models, and cover best practices for automatically validating them: What should be tested in these pipelines? How can we verify that they'll behave as we expect once in production? We’ll demonstrate how to automate tests for these scenarios and give examples using the Deepchecks Open Source testing package.
Who is this presentation for?
Data scientists, Machine Learning Engineers and Data and AI managers
Prerequisite knowledge:
Experience with training ML models
Profile
Noam is Deepchecks' Data Science lead, where he directs the development of algorithms and Machine Learning methodologies for validation of models and data. Noam holds an MSc in physics and previously served as a Data Scientist and Algorithms Researcher, developing ML and analytical models in the domain of acoustic signal processing in the IDF.