
Speaker "Ayo Adedeji" Details Back
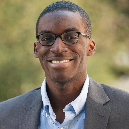

-
Name
Ayo Adedeji
-
Company
Google
-
Designation
AI Specialist
Topic
Journey from rapid Experimentation to distributed training: Develop your deep learning model for cancer diagnosis
Abstract
Bone metastasis is one of the most frequent diseases in breast, lung and prostate cancer; bone scintigraphy is the primary imaging method of screening that offers the highest sensitivity (95%) regarding metastases. To address the considerable problem of bone metastasis diagnosis, focused on breast cancer patients, artificial intelligence methods devoted to deep-learning algorithms for medical image analysis are investigated in this research work; (2) Methods: Deep learning is a powerful algorithm for automatic classification and diagnosis of medical images whereas its implementation is achieved by the use of convolutional neural networks (CNNs). The purpose of this study is to build a robust CNN model that will be able to classify images of whole-body scans in patients suffering from breast cancer, depending on whether or not they are infected by metastasis of breast cancer; (3) Results: A robust CNN architecture is selected based on CNN exploration performance for bone metastasis diagnosis using whole-body scan images, achieving a high classification accuracy of 92.50%. The best-performing CNN method is compared with other popular and well-known CNN architectures for medical imaging like ResNet50, VGG16, MobileNet, and DenseNet, reported in the literature, providing superior classification accuracy; and (4) Conclusions: Prediction results show the efficacy of the proposed deep learning approach in bone metastasis diagnosis for breast cancer patients in nuclear medicine.
Who is this presentation for?
ML Researchers, Data Scientists in Healthcare and Life Sciences
Prerequisite knowledge:
Foundational ML knowledge
What you'll learn?
Introduction to current state and future needs in the HealthCare and Life Sciences domain: a) Business and technical considerations to enable digital transformation in HCLS b) Decision process for selecting right tool for the job to accelerate time to insight and boost productivity c) Technical know-how on Vertex AI (A unified Machine Learning development platform). Vertex AI makes sophisticated AI more accessible with flexible tools for streamlined and scalable collaboration across all levels of technical expertise) d) Scientists and Researchers don’t need to be Engineers. Enhanced MLOps capabilities make practitioners job easier with robust, self-service workflow management.
Profile
I am an AI Specialist at Google that helps customers in Healthcare and Life Sciences innovate and accelerate their ML workloads through the intelligent use of cloud technology. I have an industry background in Computational Biology and an academic background in Bioengineering and Biomedical Engineering from Stanford and John Hopkins.