
Speaker "Leah Mcfarland" Details Back
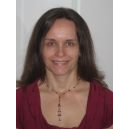

-
Name
Leah Mcfarland
-
Company
State Street
-
Designation
Product Management
Topic
Challenges in using machine learning for financial monitoring
Abstract
- Rates are still high even after initial machine learning adoption
- Historical approaches to monitoring models have been backward (eg, identify rare data pattern and impose blanket rules)
- Regulations and their application are ambiguous in not significantly distinguishing between low- and high-risk activities
- There is insufficient attention to how compliance costs serve as a barrier to access to financial services
Increasing adoption of blockchain involves a novel data processing/storage mechanism only beginning to be subjected to financial monitoring
There remains a perception that machine learning is “black box,” despite relying on well understood statistical techniques
- Poor data quality from legacy/siloed systems persists within a given institution
- Third-party proprietary and open source data need to be integrated for holistic understanding
- Semi- and unstructured data should be leveraged going forward
- There is growing expectation that data be evaluated in nearer to real-time
- Cross-border data requirements are becoming stricter
Profile
Leah McFarland is Anti-Money Laundering (AML) Transaction Monitoring Solutions Head at State Street, responsible for defining and implementing the strategy to transition from a rules-based to a features-based monitoring approach using machine learning on a big data analytics platform. Before joining State Street, she served as AML Business Solutions Head at Citibank, where she led global implementation for big data, robotic process automation, and data visualization and pioneered the use of agile project delivery. Prior to that, Leah worked at FSVC, where she managed a $5 million portfolio delivering risk management assistance to financial institutions in the Middle East, North Africa, Sub-Saharan Africa, and Eastern Europe. She began her career as a diplomat for the U.S. Department of State, serving in Moscow, the Secretary of State’s operations center in Washington, DC, and Sao Paulo. Leah has an M.A. in International Affairs from the University of California at San Diego and speaks Russian, Portuguese, Spanish, and some Mandarin Chinese.