
Speaker "Daniel Gray" Details Back
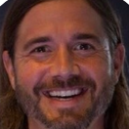

-
Name
Daniel Gray
-
Company
Atscale
-
Designation
VP Big Data
Topic
How to use a semantic layer to make AI & BI work at scale
Abstract
Learn how to bridge business intelligence and data science to make smarter data-driven decisions at scale using a semantic layer. Making data accessible to everyone within an organization is a challenge that most companies face. For example, data scientists generate forecasts and predictions while business analysts manage and drive revenue. In this session, we will cover data strategies for eliminating the silos between BI and data science teams using a semantic layer. You will get practical guidance on how to build a scalable data infrastructure and architect a strategy that supports both BI and data science programs. You will learn about: Gaining business alignment across BI and data science teams Reducing data prep for your data science & BI teams Creating governed and consistent access to features, numerics, categoricals, hierarchies, dimensions and measures Simplify and accelerate feature engineering for more powerful ML models Operationalize data science by publishing model results to BI consumers through the semantic layer Publish model outputs (e.g. forecasts, suggested actions) to broader audiences through BI tool integrations
Who is this presentation for?
Learn how to bridge business intelligence and data science to make smarter data-driven decisions at scale using a semantic layer. Making data accessible to everyone within an organization is a challenge that most companies face. For example, data scientists generate forecasts and predictions while business analysts manage and drive revenue. In this session, we will cover data strategies for eliminating the silos between BI and data science teams using a semantic layer. You will get practical guidance on how to build a scalable data infrastructure and architect a strategy that supports both BI and data science programs. You will learn about: Gaining business alignment across BI and data science teams Reducing data prep for your data science & BI teams Creating governed and consistent access to features, numerics, categoricals, hierarchies, dimensions and measures Simplify and accelerate feature engineering for more powerful ML models Operationalize data science by publishing model results to BI consumers through the semantic layer Publish model outputs (e.g. forecasts, suggested actions) to broader audiences through BI tool integrations
Prerequisite knowledge:
Basic data and analytics
What you'll learn?
The importance of a semantic layer for data & analytics
Profile
Daniel Gray | VP, Solutions Engineering, AtScale Daniel Gray brings rich experience in technical solutions engineering as well as software engineering to his work with global enterprise organizations. Prior to joining AtScale to lead the Solutions Engineering team, Daniel spent many years in the analytics space including Hewlett-Packard’s Advanced Technology Center, Vertica, and Domino Data Lab. When he’s not in the office or onsite with customers, you’ll find Daniel running, climbing, hiking, and biking – basically anything outdoors.