
Speaker "Garrett Goh" Details Back
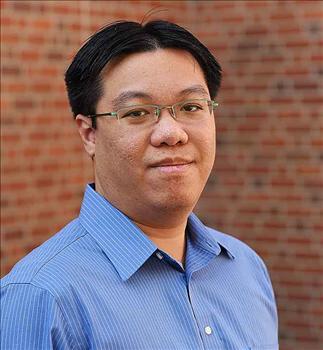
-
Name
Garrett Goh
-
Company
Pacific Northwest National Lab
-
Designation
Data Scientist
Topic
Integrating Domain Expert Knowledge into Deep Learning AI: A Case Study in Chemistry
Abstract
Beginning with the deep learning revolution in computer vision and natural language research, deep learning is now permeating at every corner of science and technology. An inherent limitation in adapting deep learning models into new domains is the necessity to train models from scratch, which is particularly time consuming, and further compounded if many different tasks are to be predicted. In this presentation, we will examine how historical research in feature-engineering by domain experts can be used to develop a deep learning “domain expert” model that can be quickly adapted to predict other tasks that it was not trained on originally. We demonstrate this example in the chemistry domain, by predicting a wide range of physical, biological, and materials properties while using only a small set of labeled data. Our results not only show better performance relative to state-of-the-art models developed using engineered features, but also improved performance relative to analogous deep learning models trained in the conventional supervised learning approach. Our findings demonstrate the potential of using “domain expert” deep neural network AI to accelerate research progress, where we envision applications not just in chemistry, but in affiliated fields, such as biotechnology, pharmaceuticals, consumer goods, materials, and potentially in other technical fields as well.