
Speaker "Sarah Wang" Details Back
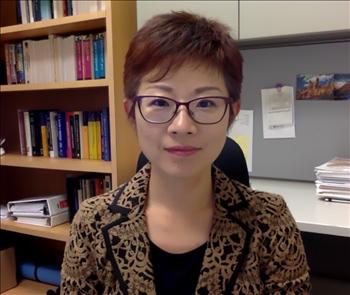
-
Name
Sarah Wang
-
Company
Caredx
-
Designation
Director
Topic
Artificial Intelligence in an Integrated Diagnostic Healthcare Ecosystem: An ImmunoOncology Use Case
Abstract
Objective: Create a multi-stakeholder ecosystem in Immuno-Oncology combining diagnostic tests, Real-World data sources and machine learning to enable a cancer risk continuum. Background: The National Academy of Sciences, Engineering and Medicine (NASEM) recently released three landmark reports that address i) rapid learning in health care with a focus on oncology, ii) the role of diagnostics for improved patient management and iii) aggregation of real-world evidence for medical product development. The NASEM reports are aligned with and describe key components for implementation for the Precision Medicine Initiative. The Precision Medicine Initiative, begun in 2015, is a long-term endeavor, involving the major governmental centers and programs with the objective to better understand and inform patient management to delay progressive chronic disease, improve the quality of life and extend patient survival while increasingly the cost-effectiveness of care using genetics and genomics. Precision Medicine has often been referred to as the strategy that enlists the right drugs for the right patients at the right time. Unfortunately, major scientific advances such as the sequencing of the human genome, Next Generation sequencing to enable broad swaths of genome to be queried in parallel, and innovative tests and services have had protracted entry into clinical practice. The primary reason for these delays is that rather than taking a directed path to translation to clinical practice to ensure timely patient access, an exploratory path is followed. Immuno-Oncology, Real-World Data and machine learning are more recent examples of discoveries that have not and will not reach many patients in time to make a difference in their care. There is a paucity of accurate noninvasive tests to query the activation status of the immune system, a lack of understanding of tumor heterogeneity (evolution) and inability to effectively extract data with requisite safety and security from real world data sources. How can the health care system change this? Methods: Biomarkers are measurable indicators of disease severity, treatment efficacy, likelihood of adverse effects and eventual clinical outcomes. For Immuno-Oncology, histological staining of tumor tissue for proteins critical for cell cycle checkpoints, the extent of the tumor mutational burden, qualitative and quantitative levels of genetic variants that inform expected efficacy or resistance to anti-cancer drugs and measurement of residual disease have been described. Not surprisingly, none of these biomarkers suffice alone to fully inform patient management. The potential to aggregate and leverage these biomarkers with optimal weighted coefficients into a score for deciding who, when and type of combined Immuno-Oncology and adjunctive treatment such as radiation or chemotherapy and when. will benefit greatly from machine learning analytics. Conclusion: The excitement of fully unsupervised deep learning with dynamic reinforcement for using Big Data sources from less characterized hospital electronic medical records with single validation studies is palpable but if health care’s focus remains there, patients will suffer. A staged approach using initially semi-supervised shallow learning, well-characterized data from carefully designed and managed registries, and multiple validation studies are essential. Without a concerted, diligent and adaptive effort to translate these molecular and computational tools to clinical practice with multiple validation studies with high level quality evidence, these medical advances will be significantly delayed. The shared investment by multiple stakeholders in improved processes and services will increase the pace of timely and effective translation of diagnostic solutions. We further need to understand that without value reimbursement, health care innovation will not advance.