
Speaker "Robert Alvarez" Details Back
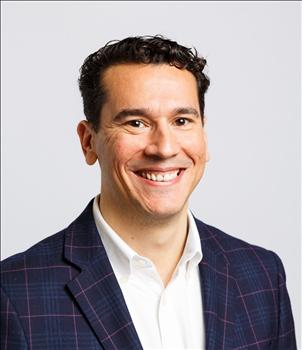
-
Name
Robert Alvarez
-
Company
Metis
-
Designation
Data Scientist
Topic
Introduction to PyTorch
Abstract
Over the past couple of years, PyTorch has been increasing in popularity in the Deep Learning community. What was initially a tool for Deep Learning researchers has been making headway in industry settings. In this session, we will cover how to create Deep Neural Networks using the PyTorch framework on a variety of examples. The material will range from beginner - understanding what is going on "under the hood", coding the layers of our networks, and implementing backpropagation - to more advanced material on RNNs, CNNs, LSTMs, & GRUs. Attendees will leave with a better understanding of the PyTorch framework. In particular, how it differs from Keras and Tensorflow. Furthermore, a link to a clean documented GitHub repo with the solutions of the examples covered will be provided.