
Speaker "Zulfiqar Ahmed" Details Back
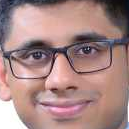

-
Name
Zulfiqar Ahmed
-
Company
REI Systems
-
Designation
Associate Data Scientist
Topic
Natural Disaster Damage Assessment from Satellite Imagery
Abstract
US is affected by severe natural disasters annually. It is important to estimate the damage quickly in order to respond with adequate measures. Current analytical bottleneck occurs due to manual review of the post-disaster areal imagery. Our goal was to develop an algorithm to detect damaged buildings in satellite images. We used semantic segmentation techniques to train custom models for buildings detection and further damage assessment. We have split the problem into building localization and roof damage detection. Custom roof damage dataset has been created containing 3,000+ images from hurricane Michael satellite images.
Profile
Zulfiqar earned his Masters degree in Computer Science from University of Washington, with his research primarily focused on Deep Learning based solutions in the cyber security industry. He collaborated with Infoblox, an IT automation and security firm, during his graduate degree to work in the Explainable AI domain. His research project titled “Interpretation of Deep Learning based Domain Generation Algorithms classifiers” focused on visualizing the feature extraction process of trained DGA classifier models currently deployed by Infoblox to provide a detailed analysis and better interpretability of neural network models utilized as DGA classifiers. During his summer internship with REI Systems, he worked on a Computer Vision based solution to assess and evaluate the damage in areas affected by natural disasters from satellite imagery. Zulfiqar's research interests include XAI, Natural Language Processing, Recommendation Systems and Computer Vision.