
Speaker "Leah Mcfarland" Details Back
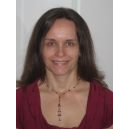

-
Name
Leah Mcfarland
-
Company
State Street
-
Designation
Product Management
Topic
Abstract
Rules-based approaches to conducting financial monitoring result in significant false positives (eg, 95%+) while potentially missing actual high risk. Most large financial institutions face significant data challenges related to legacy systems, poor data quality, missing data, and siloed data, which makes entity resolution (ie, recognizing every point the institution interacts with a given entity) difficult. New tools need to be leveraged to address these issues.
Who is this presentation for?
Anyone involved in conducting financial monitoring, or in transitioning from rules-based approaches to the use of machine learning
Prerequisite knowledge:
Familiarity with rules-based approaches to monitoring, esp. in the financial sector
What you'll learn?
Better approaches to conducting financial monitoring and leveraging available data
Profile
Leah McFarland is Anti-Money Laundering (AML) Transaction Monitoring Solutions Head at State Street, responsible for defining and implementing the strategy to transition from a rules-based to a features-based monitoring approach using machine learning on a big data analytics platform. Before joining State Street, she served as AML Business Solutions Head at Citibank, where she led global implementation for big data, robotic process automation, and data visualization and pioneered the use of agile project delivery. Prior to that, Leah worked at FSVC, where she managed a $5 million portfolio delivering risk management assistance to financial institutions in the Middle East, North Africa, Sub-Saharan Africa, and Eastern Europe. She began her career as a diplomat for the U.S. Department of State, serving in Moscow, the Secretary of State’s operations center in Washington, DC, and Sao Paulo. Leah has an M.A. in International Affairs from the University of California at San Diego and speaks Russian, Portuguese, Spanish, and some Mandarin Chinese.