
Speaker "Agus Sudjianto" Details Back
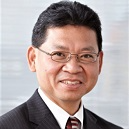

-
Name
Agus Sudjianto
-
Company
Wells Fargo Bank
-
Designation
Manager
Topic
Toward Trustworthy AI: Explainability and Robustness
Abstract
All models are wrong and when they are wrong they create financial or non-financial risks. Understanding, testing and managing model failures are the key focus of model risk management particularly model validation. Key factors of managing machine learning model risk is model explainability and robustness. Explainability is critical to evaluate conceptual soundness of models, a fundamental requirement to anticipate potential model failure when models make generalization for situation where they have not been exposed during the training. There are many explainability tools available and my focus in this talk is how to develop fundamentally interpretable self-explanatory models including Deep Learning. Since models in production will be subjected to dynamically changing environments, testing for model robustness is critical, an aspect that has been neglected in AutoML.