
Speaker "Vijayant Kumar" Details Back
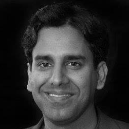

-
Name
Vijayant Kumar
-
Company
Sentient Science
-
Designation
VP Predictive Analytics
Topic
Utilizing systems-level representation to reduce prediction uncertainty of high-stakes events
Abstract
This presentation will cover our current research on a complex problem problem - how to apply systems thinking to reduce prediction uncertainty from machine learning models applied to high-consequence outcomes. The specific focus of this presentation will be on machine damage progression to catastrophic failure. Every wrong prediction about the health of a machine can either lead to a machine failure costing millions of dollars or causing an operator to stop a machine thinking it is damaged when it is not - implications of incorrect predictions for both scenarios are of high-consequence. This presentation will focus on a specific kind of large machine - wind turbines and discuss our current challenges dealing with prediction uncertainty and related adverse business outcomes from trying to do this at scale for thousands of such turbines. The presentation will discuss pitfalls, best practices and include examples of success and failures during our attempt at building a system to represent the entire system of factors which cause large machines to have damage progression and eventual failure. The presenter will try to illustrate why this is hard to build and deploy purely as data-driven machine learning models with out any representation of factors causing prediction uncertainty. Uncertainty is a less-talked-about topic in machine learning but it is critical when these algorithms directly impact high-stakes decisions in the physical world. In terms of technical content, this will touch upon topics such as signal-to-noise ratio in stochastic time-series, difference in spatial and temporal resolution of various data sources, uncertainty quantification and propagation framework (e.g. Kalman Filters) for variety of machine learning models and last but not the least, adverse impact of benign human habits - we will cover ideas that we have tried to deploy to quantify and detect these issues. The overall goal of this presentation is to (a) describe a highly complex problem of significant consequence not only economically but also on one of the greatest challenges facing us - climate change and, (b) simulate a discussion and ideas-exchange in the wider community.
Who is this presentation for?
Industrial AI executives; ML/AI practitioners; IoT practitioners; Folks interested in uncertainty quantification and cauality-based inference
Prerequisite knowledge:
None
What you'll learn?
a) How pure data-driven models are insufficient to capture diversity of conditions faced by machines in the physical world (i.e. nature) and how systems-level thinking can help build better representation systems; b) how to use predictive models to aid decision-making in high-stakes environments c) Why just loads and loads of sensor data (BigData) is insufficient by itself and needs "small" and "thick" data d)Why most industrial data should be perceived as "bad" by default unless proven otherwise.
Profile
Vijayant Kimar leads the predictive analytics team at Sentient Science and is focused on using hybrid predictive systems including data science and physics-driven modeling to provide diagnostics and prognostics through a SaaS to allow optimized predictive maintenance and life extension of wind turbines.