
Speaker "Bruno Dupire" Details Back
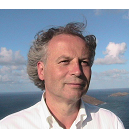

-
Name
Bruno Dupire
-
Company
Bloomberg L.P.
-
Designation
Head Of Quantitative Research
Topic
Machine Learning For Finance
Abstract
Machine Learning for Finance Bruno Dupire, Head of Quantitative Research, Bloomberg This talk explores the cross-section of machine learning and finance. I review various applications of ML in finance, both with structured data such as time series and unstructured data such as text or satellite images. I describe sentiment based strategies and how to learn optimal strategies according to the market conditions. I then present easy to use tools to perform these analyses.
Who is this presentation for?
'Finance professionals interested in applying machine learning'
Prerequisite knowledge:
Basics of finance and some machine learning
What you'll learn?
Use cases in finance where machine learning can be applied
Profile
Bruno Dupire is head of Quantitative Research at Bloomberg L.P., which he joined in 2004. Prior to this assignment in New York, he has headed the Derivatives Research teams at Société Générale, Paribas Capital Markets and Nikko Financial Products where he was a Managing Director. He is best known for having pioneered the widely used Local Volatility model (simplest extension of the Black-Scholes-Merton model to fit all option prices) in 1993 and the Functional Itô Calculus (framework for path dependency) in 2009. He is a Fellow and Adjunct Professor at NYU and he is in the Risk magazine “Hall of Fame”. He is the recipient of the 2006 “Cutting edge research” award of Wilmott Magazine and of the Risk Magazine “Lifetime Achievement” award for 2008. After a Master's degree in Artificial Intelligence in 1982 and a PhD in Numerical Analysis in 1986 he has conducted in 1987-88 a study to apply Neural Nets to time series forecasting for Caisse des Depots et Consignations. He has been applying Machine Learning to a variety of problems in Finance and has given many lectures on the topic in the Americas, Europe and Asia over the past few years.