
Speaker "Leonid Perlovsky " Details Back
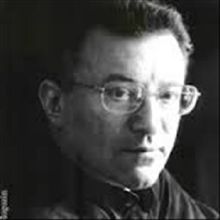
-
Name
Leonid Perlovsky
-
Company
Harvard University
-
Designation
Professor
Topic
COGNITIVE ALGORITHMS FOR BIG DATA
Abstract
Big Data problems have been efficiently addressed with cognitive algorithms modeling mechanisms of the mind. The talk describes cognitive algorithms, their applications to Big Data problems, and their foundations in mathematical models of the mind including higher cognitive abilities. Mechanisms of the mind include concepts, emotions, hierarchy, dynamic logic, and interaction between language and cognition. Big Data analytics requires algorithms modeling all these abilities. Machine learning, artificial intelligence, and modeling of the mind has been plagued by computational complexity since the 1960s. Dynamic logic overcomes computational complexity when analyzing Big Data. It is a process-logic, which replaces classical logic; it serves as a basis for cognitive algorithms and for a mathematical theory of learning, combining the mechanisms of the mind into a hierarchical system of mental processes. Each process proceeds "from vague to crisp," from vague representation-concepts to crisp ones. Brain imaging experiments (Bar et al 2006; Kveraga et al 2007) confirmed this as an adequate model of the brain perception and cognition. Computational difficulty is related to Gödelian problems in logic: computational complexity is a manifestation of Gödelian incompleteness in finite systems, such as computers or brains. The mind is "not logical." Dynamic logic overcomes this difficulty. Engineering applications demonstrate orders of magnitude improvement in Big Data analytics, data mining, information integration, financial predictions, genetic studies, cybersecurity. The talk presents the dual hierarchy model of interactions between language and cognition. It enables integrating language, text, and sensor data. A number of "mysteries" are explained: what is the difference between language and cognition; what is the role of language in cognition, why children can talk before they really understand, how much adults are different from children in this respect, etc. These are explained in the model, and explanations are confirmed in brain imaging experiments (Binder et al 2005; Price 2012). Much difficulties in developing Big Data algorithms are related to confusing language and cognition. The knowledge instinct drives acquisition of cognitive ability and is a foundation of all our higher cognitive abilities. Its satisfaction is experienced as aesthetic emotions (experimentally confirmed in Cabanac et al 2010). Efficient engineering algorithms must model these emotional abilities (Perlovsky, Deming, Ilin, 2011). The hierarchy of aesthetic emotions is discussed from understanding of everyday objects, to understanding of abstract concepts throughout the hierarchy, to the top of the mental hierarchy. Contents of these "highest" concepts are discussed and the corresponding aesthetic emotions are related to the beautiful. Experimental confirmations are discussed. Contradictions among knowledge are experienced as negative aesthetic emotions, cognitive dissonance. Development of robots and human-computer interactions require algorithms modeling this ability. Cognitive dissonance counteracts the knowledge instinct and would prevent accumulation of knowledge and the entire human evolution, if not a special ability evolved for overcoming these emotions. It follows from the dual hierarchy model that this mechanism is music. This theoretical prediction has been experimentally confirmed (Masataka et al 2012, 2013, Cabanac et al, 2013). This explains the origin and evolution of music, what Darwin called the greatest mystery.
Profile
Dr. Leonid Perlovsky is CEO, LP Information Technology, Professor of Psychology and Engineering at Northeastern Univeristy, Visiting Scholar at Harvard University, School of Engineering and Applied Sciences and Medical School Athinoula Martinos Brain Imaging Center, previously Technical Advisor and Principal Research Scientist at the AF Research Lab. He created a new area of cognitive mathematical engineering, dynamic logic, which solved a number of Big Data problems unsolvable for decades. He led research projects on mathematical models of the mind mechanisms, cognitive algorithms for integration of disparate data; interaction between cognition and language, hierarchy of abstract models, predictive and diagnostic models of culture, applications of this models to engineering and military intelligence, data mining, genetic-phenotype correlation studies, cybersecurity, commercial analytics and predictions. In leading brain imaging labs around the world his models have been proven experimentally to explain the mind mechanisms not understood previously. As Chief Scientist at Nichols Research, a $500mm high-tech DOD contractor, he led the corporate research in intelligent systems. He served as professor at Novosibirsk University and New York University; as a principal in commercial startups developing tools for biotechnology, text understanding, and financial predictions. He is invited as a keynote plenary speaker and tutorial lecturer worldwide, including most prestigious venues such as the Nobel Forum at Karolinska Institutet Stockholm; published more than 500 papers, 18 book chapters, and 7 books including “Neural Networks and Intellect,” Oxford University Press, 2001 (currently in the 3rd printing); “Emotional, Cognitive, Neural Algorithms with Engineering Applications,” Springer 2011; "Music: Passions and Cognitive Functions," Elsevier, 2016; awarded 2 patents. Dr. Perlovsky participates in organizing conferences on Computational Intelligence, Chair for the IEEE Task Force on The Mind and Brain, on the International Neural Network Society (INNS) Board of Governors, past Chair for the IEEE Boston Computational Intelligence Chapter; INNS Chair of The Award Committee. He serves on the Editorial Board of ten professional journals, has founded and serves as Editor-in-Chief for “Physics of Life Reviews,” the IF=8.2, ranked #3 in the world by Thomson Reuters among 72 journals in biophysics. He received National and International awards including The Best Paper Award at Russian most prestigious magazine, Zvezda; the Gabor Award, the top engineering award from the INNS; and the John McLucas Award, the highest US Air Force Award for basic research.