
Speaker "Halim Abbas" Details Back
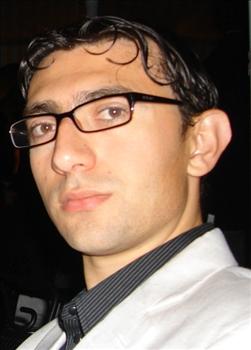

-
Name
Halim Abbas
-
Company
Think Big
-
Designation
Chief Data Scientist
Topic
Thinking Big and Small - How Bigger is not Always Better
Abstract
Recent leaps in Big Data technology have rendered technologists across industries hungry for crunching evermore data at unprecedented scale. But data science tasks in general (and statistical/predictive modeling in particular) often don’t benefit from incorporating massive amounts of data beyond a certain limit. Worse still, in some contexts the scale of data can actually be detrimental to certain data science activities. This talk uses real life examples as well as tailored modeling experiments in order to bring into light data science contexts where adding more data could result in more hindrance than benefit.
Profile
Halim is a high tech innovator who spearheaded world-class data science projects at game changing tech firms such as eBay and Quixey. Formally educated in Machine Learning, his professional expertise span Information Retrieval, Natural Language Processing, and modeling with Big Data. Outside work, Halim is keen on reading and writing about social and natural history, paleontology, and cognitive sciences.