
Speaker "Erik Schmidt" Details Back
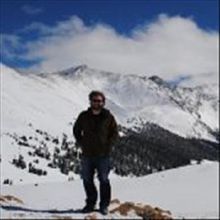
-
Name
Erik Schmidt
-
Company
Pandora
-
Designation
Senior Data Scientist
Topic
Machine Listening at Pandora
Abstract
Finding the music of the moment can often be a challenging problem even for humans with well-versed musical tastes. These challenges further explode into myriad complexities when attempting to construct algorithmic approaches for automatic playlist generation. A variety of factors play a role in influencing a listeners perception of what music is appropriate on a given seed (e.g., musicological, social, geographical, generational), and these factors vary across different contexts and listeners. Furthermore, as opposed to more traditional recommender systems which need only to recommend a single item or set of items, Pandora’s recommenders must provide an evolving set of sequential items which constantly keep the experience new and exciting. The talk will present an overview of recommendation at Pandora, followed by a deep dive into the development of powerful machine listening systems which leverage the vast data resources of the MGP. I will discuss the extraction of acoustic feature representations, supervised machine learning, and additionally provide some insights into the application of listener feedback to develop powerful content-based recommender systems.