
Speaker "Anand Oka" Details Back
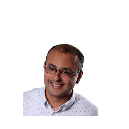

-
Name
Anand Oka
-
Company
Microsoft
-
Designation
Director
Topic
Reinforcement Learning in Business Applications
Abstract
Over the past decade we have seen an increasing use of Artificial Intelligence (AI) in business decision making, with the goal of higher decision accuracy and better business outcomes. Many business problems are “open loop” meaning that the decisions made by the ML model do not affect the observations that occur in the future. Canonical unsupervised and supervised learning are eminently suited to solving such problems. However, there is also a large class of “closed loop” problems where the decisions immediately and appreciably change the state of the business environment and hence modify its future behavior. Examples include choosing pricing and promotions, fraud management and supply chain management. In general, for predicting good decisions in such closed-loop processes we need to account for our past decisions and their rewards. In such situations the machinery of unsupervised and supervised learning is found insufficient. Classically such “closed-loop” problems in business have been solved through the Planning, which involves a two-step process – first learn the model of the environment and then solve a well-defined optimization problem to recommend a plan of action given current observations and the known environment model. The problem with this approach is that if the model of the environment undergoes frequent and large systemic “shocks”, there is insufficient time and data to ever learn the model accurately. This means that planning relies on a perpetually biased model and no matter how well the subsequent optimization problem is solved the rewards earned will not be maximized. Additionally, re-estimation of the environment model frequently can also be computationally very costly. Therefore we look to a new type of machine learning called Reinforcement Learning (RL) which seems to be the suitable ML technology for artificially intelligent decision making in closed-loop business applications where systemic shocks are large and frequent. Being “model-free”, it moves away from the classical two-step planning process and embraces continuous update of decision policies that entirely bypass the need for an environment model. This gives it better agility as well as robustness relative to classical planning. We illustrate the utility of RL in fraud protection for online/ecommerce, where it can help answer the question “How should we continuously tune the rejection threshold for the fraud score to maximize long term profit?”.