
Speaker "Keisuke Inoue" Details Back
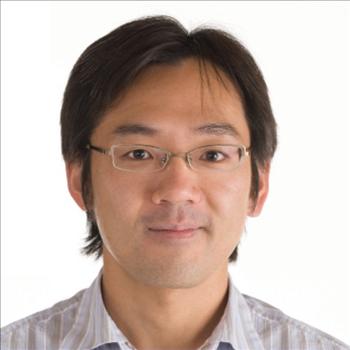
-
Name
Keisuke Inoue
-
Company
Emogi Technologies, Inc
-
Designation
VP Data Science
Topic
Conversation Graph: Understanding Consumer Sentiment, Messaging Topics, and Content Sharing
Abstract
The goal of this research initiative is to build a technology that optimally suggests relevant, fun, and unique content (i.e. contextual stickers and GIFs) to messaging apps users to be shared within their peer-to-peer conversations. The Conversation Graph processes the consumer sentiment, messaging topics, and content sharing behaviors. This research expands and utilizes the sentiment analysis, machine learning, and natural language processing work that was previously presented by Keisuke at the 2017 Sentiment Symposium. The talk will include: 1) an overview of the data architecture of the Conversation Graph -- how the data is collected, processed and analyzed, 2) a case study analyzing the context and reactions to content sharing on messaging apps and 3) an experiment to build a predictive model of content sharing based on the users' behavioral and contextual data. The research and case study is based on the data that has been collected via Emogi’s partnerships with various mobile application partners such as Whisper, TextNow, TextPlus, and more. Content data revolves around the original emoji, stickers and GIFs created by the Emogi Content Studio, including branded examples for leading brands like McDonald's, IKEA, Universal Pictures, Moet Hennessy among others. The goal of this research initiative is to build a technology that optimally suggests relevant, fun, and unique content (i.e. contextual stickers and GIFs) to messaging apps users to be shared within their peer-to-peer conversations. The Conversation Graph processes the consumer sentiment, messaging topics, and content sharing behaviors. The talk will include: 1) an overview of the data architecture of the Conversation Graph -- how the data is collected, processed and analyzed, 2) a case study analyzing the context and reactions to content sharing on messaging apps and 3) an experiment to build a predictive model of content sharing based on the users' behavioral and contextual data. The research and case study is based on the data that has been collected via Emogi’s partnerships with various mobile application partners such as Whisper, TextNow, TextPlus, and more. Content data revolves around the original emoji, stickers and GIFs created by the Emogi Content Studio, including branded examples for leading brands like McDonald's, IKEA, Universal Pictures, Moet Hennessy among others.