
Speaker "Shalini Ghosh" Details Back
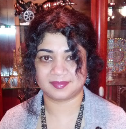

-
Name
Shalini Ghosh
-
Company
Amazon
-
Designation
Principal
Topic
Multimodal Machine Learning for Video and Image Analysis
Abstract
In this talk, we will first discuss multimodal ML for video content analysis. Videos typically have data in multiple modalities like audio, video, and text (captions). Understanding and modeling the interaction between different modalities is key for video analysis tasks like categorization, object detection, activity recognition, etc. However, data modalities are not always correlated -- so, learning when modalities are correlated and using that to guide the influence of one modality on the other is crucial. Another salient feature of videos is the coherence between successive frames due to continuity of video and audio, a property that we refer to as temporal coherence. We show how using non-linear guided cross-modal signals and temporal coherence can improve the performance of multimodal ML models for video analysis tasks like categorization. We also created a hierarchical taxonomy of categories internally. Our experiments on the large-scale YouTube-8M dataset show how our approach significantly outperforms state-of-the-art multimodal ML model for video categorization using our taxonomy, as well as generalizes well to an internal dataset of video segments from actual TV programs. The next part of the talk will briefly discuss our work on visual dialog and explainability of multimodal ML models. We will conclude the talk by outlining potential applications of multimodal ML to several applications.
Who is this presentation for?
For audience interested in AI innovation
Prerequisite knowledge:
Familiarity with basic knowledge of deep learning
What you'll learn?
The state of the art in the field of multimodal AI research, especially in the conversational AI space
Profile
Dr. Shalini Ghosh is a Principal Research Scientist at Amazon Science (Alexa). Her main area of research interest is machine learning/deep learning with applications to various domains (e.g., natural language, speech, computer vision, multimodal AI, security, trustworthy systems). Previously, Dr. Ghosh was a Director/Principal Scientist in Samsung Research America for 2+ years, where she led an R&D team for video understanding in Samsung Smart TV. Prior to that, Dr. Ghosh was a Principal Scientist at SRI International where she worked for 12+ years on applying machine learning to a broad set of domains. She was also a Visiting Scientist at Google Research, where she worked with the Google Brain team on developing contextual language models (e.g., contextual LSTMs) for large-scale language understanding and dialog modeling. Dr. Ghosh got her PhD from the University of Texas at Austin. She serves as Area Chair for multiple ML conferences (e.g., ICLR, ICML), and her research has won a Best Paper award and a Best Student Paper Runner-up award for applications of ML to dependable computing. She was selected as one of the “30 Influential Women Advancing AI” in 2019, and her ReWork talk was selected as one of the “Top 5 AI talks” in 2020. More information about her work can be found at http://shalinighosh.com/.