
Speaker "Ahmed Bakhaty" Details Back
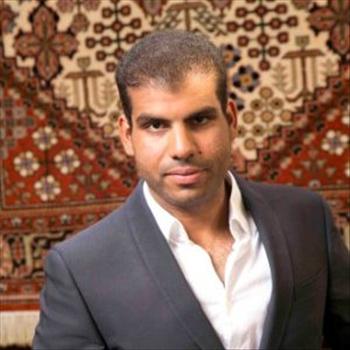
-
Name
Ahmed Bakhaty
-
Company
CBJ Global
-
Designation
Research Engineer
Topic
Filling in Missing Data: Matrix Completion
Abstract
In practice, missing data is a rampant issue, particularly in frontier spaces that make use of high-dimensional data. The simplest way to remedy the issue is to fill in missing entries with statistics like mean or median; more intelligent methods include expectation-maximization and multiple imputations, though these methods are not quite scalable. The compressed-sensing literature offers a solution to this problem via matrix completion, which guarantees to recover missing data exactly given only a small number of sampled entries. Matrix completion is both scalable and, as recently shown in a paper, guaranteed to converge to a globally-optimal solution. In this talk, we will review the original matrix completion algorithm, several improvements and variations, and provide applications.