
Speaker "Miguel Campo" Details Back
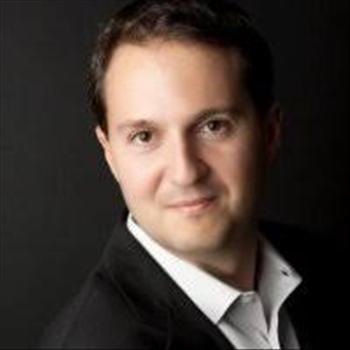
-
Name
Miguel Campo
-
Company
Fox
-
Designation
SVP
Topic
AI and the Power of Storytelling
Abstract
Recommendation systems for movie theatrical releases are emerging machine learning tools used for greenlighting de- cisions, movie positioning studies, and marketing and dis- tribution. User level prediction, especially for mid-budget movies, is a key component of a successful multichannel dis- tribution strategy (e.g. theater and streaming). Prediction is a challenging problem due to the inherent di culty of modeling movies that have not been made, the zero-sum nature of competition, the distributed nature of the data ecosystem (which goes back to 1948’s U.S. Supreme Court verdict forcing studios to divest from movie theater chains), and the di culty of capturing cash transactions at the box o ce, esp. for younger audiences. Prediction for frequent moviegoers, who bring a larger share of the theatrical rev- enues, is even harder as heavy moviegoers not only see more movies but also have a taste for di↵erent kind of movies. In the paper, we present a theatrical release recommendation sys- tem used in real-world studio business decisions. We propose a novel hybrid Collaborative Filtering (CF) model that cap- tures the features of the movie synopsis, and combines them with attendance and demographic data to enable accurate in-matrix and cold-start recommendations. We evaluate the system using a large-scale dataset and observe up to 12.2% improvement in AUC over various baseline algorithms. We show how the system is used to assist real-world decision making, and discuss the unique idiosyncrasies of moviegoers and their impact on recommendation systems.
Profile
Miguel Campo is SVP of Data Science & Analytics at Twentieth Century Fox Film Corp. Miguel’s expertise is at the intersection of machine learning and signal processing. At Fox, he heads up the team developing movie recommendation systems, script and video analysis tools, and media measurement tools. Prior to Fox, Miguel led data science groups at EY Advisory and Disney Science. Miguel has a MSEE, a PhD in Information Systems from NYU Stern and a Post-Doc from Dartmouth College. He holds a number of patents in algorithmic modeling and has published in peer review journals. He lives in LA with his wife and two daughters, and in his free time enjoys trail running and surfing.