
Speaker "Sanhita Sarkar" Details Back
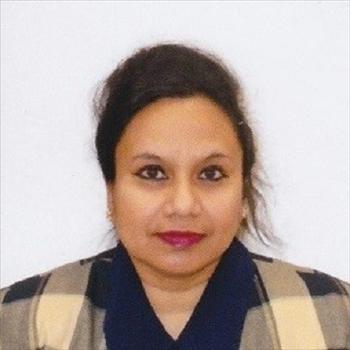

-
Name
Sanhita Sarkar
-
Company
Western Digital
-
Designation
Director Engineering
Topic
Building Intelligent AI Data Pipelines for Improved Data Center Economics
Abstract
Profile
Sanhita Sarkar is a Global Director, Software Development, Data Center Architectures & Ecosystems, at Western Digital, where she focuses on software design and development of analytics features and solutions spanning edge, data center, data lake, and cloud. She has expertise in key vertical markets such as the Industrial Internet of Things (IIoT), Defense and Intelligence, Financial Services, Genomics, and Healthcare. Sanhita previously worked at Teradata, SGI, Oracle, and a few startups. She was responsible for overseeing design, development, and delivery of optimized software and solutions involving large memory, scale-up, and scale-out systems. Sanhita has authored multiple patents, published several papers, and has spoken at several conferences and meetups. She received her Ph.D. in Electrical Engineering and Computer Science from the University of Minnesota, Minneapolis.