
Speaker "Oriol Nieto" Details Back
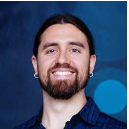

-
Name
Oriol Nieto
-
Company
Pandora
-
Designation
Senior Scientist
Topic
Recommending Music with Waveform-based Architectures
Abstract
In this talk we discuss deep models that use waveform representations as input to estimate an embedded space where distances become meaningful when recommending music. Such space is initially produced by analyzing listener behavior, thus becoming a powerful tool to recommend the most popular content in a given music catalog but weak in terms of addressing the so-called "cold start problem," i.e., to recommend content that has spun infrequently or never at all and thus, has little listener behavior data associated with it. We show how, given enough data, deep waveform-based architectures [1] can estimate such spaces more accurately than spectrogram-based ones. Moreover, by using other sources of data (e.g., human labeled music attributes such as the ones in the Music Genome Project) with late-fusion multimodal networks [2], we achieve higher accuracy when predicting these embedded spaces. Finally, several musical examples are explored to further illustrate the recommendation results.
Profile
Oriol Nieto, born in Barcelona in 1983, is a data scientist at Pandora. He obtained his Ph.D in Music Data Science from the Music and Audio Research Lab at NYU (New York, NY, USA) in 2015. He holds an M.A. in Music, Science and Technology from Stanford University (Stanford, CA, USA), an M.Sc in Information Technologies from Pompeu Fabra University (Barcelona, Spain), and a B.Sc. in Computer Science from Polytechnic University of Catalonia (Barcelona, Spain). His research focuses on topics such as music information retrieval, large scale recommendation systems, and machine learning with especial emphasis on deep architectures. He plays guitar, violin, and sings (and screams) in his spare time.