
Speaker "Chakri Cherukuri" Details Back
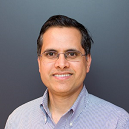

-
Name
Chakri Cherukuri
-
Company
Bloomberg LP
-
Designation
Senior Researcher
Topic
Applied Machine Learning In Finance
Abstract
Quantitative finance is a rich field in finance where advanced mathematical and statistical techniques are employed by both sell-side and buy-side institutions. Techniques like time series analysis, stochastic calculus, multivariate statistics, and numerical optimization are often used by "quants” for modeling asset prices, portfolio construction and optimization, and building automated trading strategies. Chakri Cherukuri demonstrates how to apply machine learning techniques in quantitative finance, covering use cases involving both structured and alternative datasets. The focus of the talk will be on promoting reproducible research and building interpretable models.
Profile
Chakri Cherukuri is a senior researcher in the Quantitative Financial Research group at Bloomberg LP. His research interests include quantitative portfolio management, algorithmic trading strategies and applied machine learning/deep learning. Previously, he built analytical tools for the trading desks at Goldman Sachs and Lehman Brothers. Before that he worked in the Silicon Valley for startups building enterprise software applications. He has extensive experience in scientific computing and software development. He is a core contributor to bqplot, a 2D plotting library for the Jupyter notebook. He holds an undergraduate degree in mechanical engineering from Indian Institute of Technology (IIT), Madras, and an MS in computational finance from Carnegie Mellon University.