
Speaker "Pei-Yun S. Hsueh" Details Back
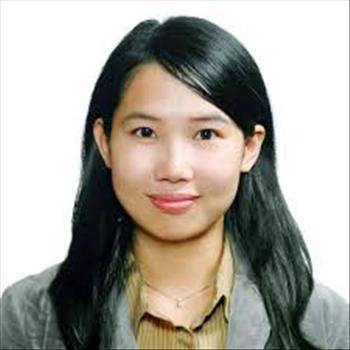
-
Name
Pei-Yun S. Hsueh
-
Company
IBM
-
Designation
Analytics Team Lead
Topic
Use cases of Interpretable AI in Healthcare: Transforming population evidence to precision health application with patient-generated health data
Abstract
The rise of consumer awareness and the prevalence of care management and wearables and sensor technologies have enabled the accumulation of personal health data outside of clinical settings and offered promise to precision health applications. Making individual recommendations based on the accumulated personal health data, however, incurs multi-level challenges. These include applying population-level evidence at an individual level and inducing actionable feedback from sequential observations at each potential touchpoint in the intermediate stages. The goal of this talk is to introduce vertical industry use cases to support the development of an interpretable AI framework that can help capture individual predictive pathways from the observed behaviors and proxy outcomes. For example, the observations of past exercise-stress behavioral pathways could be used to inform decision of physical activity recommendations. As a result, a new generation of AI methods, including reinforcement learning, are developed to incorporate the notion of explainability into the learning process. Advanced techniques and promising applications in practice have been implemented to combine a priori knowledge with cognitive data analysis and decision support in healthcare. Traditionally, health data analysis focuses on directly learning patterns from data as examples. However, humans learn not only from examples, but also from various knowledge sources and interactions. This is especially important in the healthcare domain, where deriving highly interpretable clinical decisions from high-level knowledge bases such as guidelines is the norm. The interpretable AI framework that incorporate knowledge learning symbiosis entail promising applications in healthcare practice, e.g., creating problem-specific tools to couple knowledge with patient data to generate interpretable real-world evidence. In this talk, we will review the recent progress in the field and report on the gaps and challenges identified in the use cases.
Profile
Pei-Yun S. Hsueh is currently IBM Academy of Technology Member and Health Behavioral Insights lead in the Center for Computational Health at IBM T.J. Watson Research Center. She has been a pioneer in the area of behavioral analytics and consumer and pervasive health informatics. She is leading the efforts of building a cognitive learning framework for precision health based on patient-generated health data, including user interaction for cognitive care management, ecological momentary assessments and mobile/wearable/IOT health data. She received her master's degree from University of California, Berkeley and PhD in Informatics from University of Edinburgh respectively. She is a regular winner of IBM Inventor awards, Manager Choice Awards and IBM Eminence Award. She authored 20+ patents, 40+ technical papers, and 3 textbook chapters. She is currently serving on the Scientific Program Committee of American Medical Informatics Association Annual Symposium 2017 and the leadership of Consumer and Pervasive Informatics Work Group. Her expertise in the emerging research fields makes her a sought-after speaker and consultant in vertical industry solutions. Prior to IBM, she has served as the European Google Anita Borg Scholar and worked in EU FP projects with 22 partner sites across 7 countries.