
Speaker "Nikhil Aggarwal" Details Back
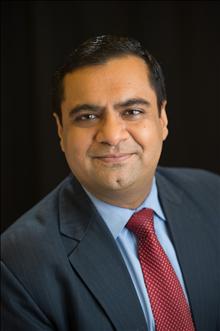
-
Name
Nikhil Aggarwal
-
Company
Ivalley Innovation Center
-
Designation
Fintech Entrepreneur In Residence
Topic
Demystifying the Intersection between Financial Crime Risk and Machine Learning
Abstract
Financial Crime Risk is the adverse financial, reputational or regulatory impact from the breach of anti-money laundering, economic sanctions, anti-bribery and corruption laws and regulations. Continued regulatory scrutiny and enforcement has resulted in financial institutions bolstering their controls framework and capabilities. Data mining and machine learning play a core role in delineating and quantifying risk; and making informed policy and control decisions to operate within the financial instruction’s risk appetite. This session outlines multiple use-cases of data mining and machine learning across the “customer” and “transaction” lenses. Insights generated will result in improved risk classification, a more calibrated risk appetite statement, and mitigation of criminal behavior.
Profile
Nikhil has 18 years of international experience spanning Consumer and Corporate Banking across financial crime compliance, fraud, operations, credit risk, user experience, sales and marketing analytics. He has built and led global teams specializing in data management, metrics design and dashboards, visualization, data mining, statistical modeling and financial modeling. He is currently advising start ups in the RegTech and FinTech space at the iValley Innovation Center, a co-creation studio that helps build and launch FinTech startups. Nikhil has held analytics positions with global institutions including Standard Chartered Bank, Bank of America, Citigroup and Capital One.