
Speaker "Liangjie Hong" Details Back
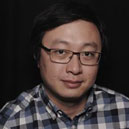
-
Name
Liangjie Hong
-
Company
Etsy
-
Designation
Head Of Data Science
Topic
A Gradient-based Adaptive Learning Framework for Efficient Personal Recommendation
Abstract
Recommending personalized content to users is a long-standing challenge to many online services including Facebook, Yahoo, LinekdIn and Twier. Traditional recommendation models such as latent factor models and feature-based models are usually trained for all users and optimize an “average” experience for them, yielding sub-optimal solutions. Although multi-task learning provides an opportunity to learn personalized models per user, learning algorithms are usually tailored to specific models (e.g., generalized linear model, matrix factorization and etc.), creating obstacles for a unied engineering interface, which is important for large Internet companies. In this paper, we present an empirical framework to learn user-specific personal models for content recommendation by utilizing gradient information from a global model. Our proposed method can potentially benet any model that can be optimized through gradients, offering a lightweight yet generic alternative to conventional multi-task learning algorithms for user personalization. We demonstrate the effectiveness of the proposed framework by incorporating it in three popular machine learning algorithms including logistic regression, gradient boosting decision tree and matrix factorization. Our extensive empirical evaluation shows that the proposed framework can significantly improve the efficiency of personalized recommendation in real-world datasets.
Profile
I am Head of Data Science at Etsy Inc., managing a group of data scientists to deliver cutting-edge scientific solutions for: * Search and Discovery * Personalization and Recommendation * Computational Advertising * Deep Learning and Image Understanding Previously, I was Senior Manager of Research at Yahoo Research from 2013 to 2016, leading science efforts for Personalization and Search Sciences. Our team helped to drive science solutions for: Yahoo Homepage News Streams, Yahoo Aviate App Recommendation, Yahoo Tumblr Blog Recommendation, Yahoo Video Recommendation, Yahoo Assistant/Bot Platform and Yahoo Mobile Search. Over the past several years, I have published papers in all major international conferences in data mining, machine learning and information retrieval, such as SIGIR, WWW, KDD, CIKM, AAAI, WSDM, RecSys and ICML with more than 1,700 citations (H-index: 17), with WWW 2011 Best Poster Paper Award, WSDM 2013 Best Paper Nominated and RecSys 2014 Best Paper Award. I have served as program committee members in KDD, WWW, SIGIR, WSDM, AAAI, EMNLP, ICWSM, ACL, CIKM, IJCAI and several workshops. I have helped to review articles in top journals. I have co-founded User Engagement Optimization Workshop, which has been held in conjunction with CIKM 2013 and with KDD 2014.