
Speaker "Sujay Kakarmath" Details Back
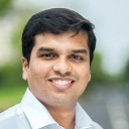

-
Name
Sujay Kakarmath
-
Company
Harvard Medical School
-
Designation
Chief Scientist
Topic
Importance of clinical context in evaluating machine learning-based solutions for healthcare
Abstract
Novel predictive algorithms developed using machine learning methods are often optimized to achieve the best area under the receiver operating characteristic curve (AUC). However, this metric is often not relevant clinically. How, then, can health professionals make conclusions about the real utility of an algorithm? The Algorithm Science team at Partners Connected Health invests a great deal of time thinking about the right questions, working out potential pitfalls and developing best practices in evaluating machine learning-based solutions.
Who is this presentation for?
Business leaders and clinical end-users of machine learning and AI solutions
Prerequisite knowledge:
None
What you'll learn?
This presentation will provide the audience with a better understanding of how to best gauge the clinical utility of an algorithm.