
Speaker "Daniel Shenfeld" Details Back
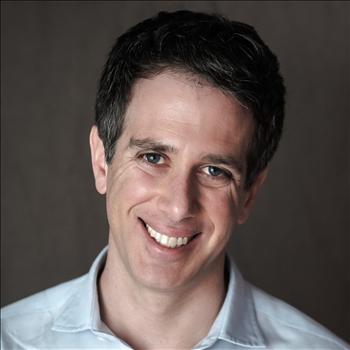
-
Name
Daniel Shenfeld
-
Company
Manganese Solutions
-
Designation
Principal
Topic
Product/Data Fit: The Lean Startup Method and AI Products
Abstract
Introducing machine learning and AI components into a product leads to strategic product development challenges, arising from the inherent uncertainty involved in data and machine learning models. How to quantify and measure the value of data? How to clearly define data science deliverables? When should an unsuccessful modeling effort lead to a product pivot? This talk aims to provide a unifying framework to tackle these challenges. I will introduce the concept of product/data fit and explain how it relates to product/market fit. I will describe how product considerations determine prediction value and guide the choice of modeling metrics, and how the lean startup build-measure-learn methodology can be adapted to accelerate both product/market fit and product/data fit. I will discuss case studies from healthcare and other verticals, highlighting guiding principles and common pitfalls and demonstrating how this approach can shorten time to market and help achieve financial business goals of AI driven products.