
Speaker "Neil Tenenholtz" Details Back
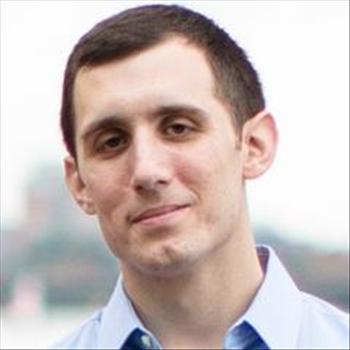
-
Name
Neil Tenenholtz
-
Company
Fitbit
-
Designation
Data Scientist
Topic
Regression in the Wild: Solving the Challenges Posed by Dirty Data
Abstract
Real-world data is often dirty and filled with physically implausible data points. Vanilla regression techniques can often fail catastrophically in the presence of such outliers and points of high leverage. While cleaning the data can be effective, it becomes challenging as the size of the data set grows. Robust model fitting techniques solve many of these problems by limiting the influence of these outliers. This talk will focus on a few of these robust fitting techniques, including RANSAC, m-estimators, and quantile regression.
Profile
Summa cum laude from University of Pennsylvania with degrees in Mechanical Enginering, Mathematics, and Finance. Masters in Robotics from University of Pennsylania. PhD from Harvard University focusing on fast surgical simulation of heart surgery. Previously a team lead and research scientist at a Vecna Technologies (a robotics company in Cambridge, MA) where he applied machine learning to human-robot interaction. Now a research scientist at Fitbit. More detailed bio available upon request.